mirror of
https://github.com/tailscale/tailscale.git
synced 2024-11-25 19:15:34 +00:00
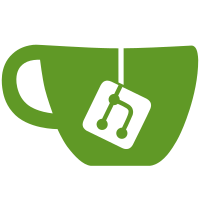
Updates #11058 Change-Id: I35e7ef9b90e83cac04ca93fd964ad00ed5b48430 Signed-off-by: Brad Fitzpatrick <bradfitz@tailscale.com>
262 lines
7.6 KiB
Go
262 lines
7.6 KiB
Go
// Copyright (c) Tailscale Inc & AUTHORS
|
||
// SPDX-License-Identifier: BSD-3-Clause
|
||
|
||
// Package topk defines a count-min sketch and a cheap probabilistic top-K data
|
||
// structure that uses the count-min sketch to track the top K items in
|
||
// constant memory and O(log(k)) time.
|
||
package topk
|
||
|
||
import (
|
||
"container/heap"
|
||
"hash/maphash"
|
||
"math"
|
||
"slices"
|
||
"sync"
|
||
)
|
||
|
||
// TopK is a probabilistic counter of the top K items, using a count-min sketch
|
||
// to keep track of item counts and a heap to track the top K of them.
|
||
type TopK[T any] struct {
|
||
heap minHeap[T]
|
||
k int
|
||
sf SerializeFunc[T]
|
||
cms CountMinSketch
|
||
}
|
||
|
||
// HashFunc is responsible for providing a []byte serialization of a value,
|
||
// appended to the provided byte slice. This is used for hashing the value when
|
||
// adding to a CountMinSketch.
|
||
type SerializeFunc[T any] func([]byte, T) []byte
|
||
|
||
// New creates a new TopK that stores k values. Parameters for the underlying
|
||
// count-min sketch are chosen for a 0.1% error rate and a 0.1% probability of
|
||
// error.
|
||
func New[T any](k int, sf SerializeFunc[T]) *TopK[T] {
|
||
hashes, buckets := PickParams(0.001, 0.001)
|
||
return NewWithParams(k, sf, hashes, buckets)
|
||
}
|
||
|
||
// NewWithParams creates a new TopK that stores k values, and additionally
|
||
// allows customizing the parameters for the underlying count-min sketch.
|
||
func NewWithParams[T any](k int, sf SerializeFunc[T], numHashes, numCols int) *TopK[T] {
|
||
ret := &TopK[T]{
|
||
heap: make(minHeap[T], 0, k),
|
||
k: k,
|
||
sf: sf,
|
||
}
|
||
ret.cms.init(numHashes, numCols)
|
||
return ret
|
||
}
|
||
|
||
// Add calls AddN(val, 1).
|
||
func (tk *TopK[T]) Add(val T) uint64 {
|
||
return tk.AddN(val, 1)
|
||
}
|
||
|
||
var hashPool = &sync.Pool{
|
||
New: func() any {
|
||
buf := make([]byte, 0, 128)
|
||
return &buf
|
||
},
|
||
}
|
||
|
||
// AddN adds the given item to the set with the provided count, returning the
|
||
// new estimated count.
|
||
func (tk *TopK[T]) AddN(val T, count uint64) uint64 {
|
||
buf := hashPool.Get().(*[]byte)
|
||
defer hashPool.Put(buf)
|
||
ser := tk.sf((*buf)[:0], val)
|
||
|
||
vcount := tk.cms.AddN(ser, count)
|
||
|
||
// If we don't have a full heap, just push it.
|
||
if len(tk.heap) < tk.k {
|
||
heap.Push(&tk.heap, mhValue[T]{
|
||
count: vcount,
|
||
val: val,
|
||
})
|
||
return vcount
|
||
}
|
||
|
||
// If this item's count surpasses the heap's minimum, update the heap.
|
||
if vcount > tk.heap[0].count {
|
||
tk.heap[0] = mhValue[T]{
|
||
count: vcount,
|
||
val: val,
|
||
}
|
||
heap.Fix(&tk.heap, 0)
|
||
}
|
||
return vcount
|
||
}
|
||
|
||
// Top returns the estimated top K items as stored by this TopK.
|
||
func (tk *TopK[T]) Top() []T {
|
||
ret := make([]T, 0, tk.k)
|
||
for _, item := range tk.heap {
|
||
ret = append(ret, item.val)
|
||
}
|
||
return ret
|
||
}
|
||
|
||
// AppendTop appends the estimated top K items as stored by this TopK to the
|
||
// provided slice, allocating only if the slice does not have enough capacity
|
||
// to store all items. The provided slice can be nil.
|
||
func (tk *TopK[T]) AppendTop(sl []T) []T {
|
||
sl = slices.Grow(sl, tk.k)
|
||
for _, item := range tk.heap {
|
||
sl = append(sl, item.val)
|
||
}
|
||
return sl
|
||
}
|
||
|
||
// CountMinSketch implements a count-min sketch, a probabilistic data structure
|
||
// that tracks the frequency of events in a stream of data.
|
||
//
|
||
// See: https://en.wikipedia.org/wiki/Count%E2%80%93min_sketch
|
||
type CountMinSketch struct {
|
||
hashes []maphash.Seed
|
||
nbuckets int
|
||
matrix []uint64
|
||
}
|
||
|
||
// NewCountMinSketch creates a new CountMinSketch with the provided number of
|
||
// hashes and buckets. Hashes and buckets are often called "depth" and "width",
|
||
// or "d" and "w", respectively.
|
||
func NewCountMinSketch(hashes, buckets int) *CountMinSketch {
|
||
ret := &CountMinSketch{}
|
||
ret.init(hashes, buckets)
|
||
return ret
|
||
}
|
||
|
||
// PickParams provides good parameters for 'hashes' and 'buckets' when
|
||
// constructing a CountMinSketch, given an estimated total number of counts
|
||
// (i.e. the sum of all counts ever stored), the error factor ϵ as a float
|
||
// (e.g. 1% is 0.001), and the probability factor δ.
|
||
//
|
||
// Parameters are chosen such that with a probability of 1−δ, the error is at
|
||
// most ϵ∗totalCount. Or, in other words: if N is the true count of an event,
|
||
// E is the estimate given by a sketch and T the total count of items in the
|
||
// sketch, E ≤ N + T*ϵ with probability (1 - δ).
|
||
func PickParams(err, probability float64) (hashes, buckets int) {
|
||
d := math.Ceil(math.Log(1 / probability))
|
||
w := math.Ceil(math.E / err)
|
||
|
||
return int(d), int(w)
|
||
}
|
||
|
||
func (cms *CountMinSketch) init(hashes, buckets int) {
|
||
for range hashes {
|
||
cms.hashes = append(cms.hashes, maphash.MakeSeed())
|
||
}
|
||
|
||
// Need a matrix of hashes * buckets to store counts
|
||
cms.nbuckets = buckets
|
||
cms.matrix = make([]uint64, hashes*buckets)
|
||
}
|
||
|
||
// Add calls AddN(val, 1).
|
||
func (cms *CountMinSketch) Add(val []byte) uint64 {
|
||
return cms.AddN(val, 1)
|
||
}
|
||
|
||
// AddN increments the count for the given value by the provided count,
|
||
// returning the new count.
|
||
func (cms *CountMinSketch) AddN(val []byte, count uint64) uint64 {
|
||
var (
|
||
mh maphash.Hash
|
||
ret uint64 = math.MaxUint64
|
||
)
|
||
for i, seed := range cms.hashes {
|
||
mh.SetSeed(seed)
|
||
|
||
// Generate a hash for this value using Lemire's alternative to modular reduction:
|
||
// https://lemire.me/blog/2016/06/27/a-fast-alternative-to-the-modulo-reduction/
|
||
mh.Write(val)
|
||
hash := mh.Sum64()
|
||
hash = multiplyHigh64(hash, uint64(cms.nbuckets))
|
||
|
||
// The index in our matrix is (i * buckets) to move "down" i
|
||
// rows in our matrix to the row for this hash, plus 'hash' to
|
||
// move inside this row.
|
||
idx := (i * cms.nbuckets) + int(hash)
|
||
|
||
// Add to this row
|
||
cms.matrix[idx] += count
|
||
ret = min(ret, cms.matrix[idx])
|
||
}
|
||
return ret
|
||
}
|
||
|
||
// Get returns the count for the provided value.
|
||
func (cms *CountMinSketch) Get(val []byte) uint64 {
|
||
var (
|
||
mh maphash.Hash
|
||
ret uint64 = math.MaxUint64
|
||
)
|
||
for i, seed := range cms.hashes {
|
||
mh.SetSeed(seed)
|
||
|
||
// Generate a hash for this value using Lemire's alternative to modular reduction:
|
||
// https://lemire.me/blog/2016/06/27/a-fast-alternative-to-the-modulo-reduction/
|
||
mh.Write(val)
|
||
hash := mh.Sum64()
|
||
hash = multiplyHigh64(hash, uint64(cms.nbuckets))
|
||
|
||
// The index in our matrix is (i * buckets) to move "down" i
|
||
// rows in our matrix to the row for this hash, plus 'hash' to
|
||
// move inside this row.
|
||
idx := (i * cms.nbuckets) + int(hash)
|
||
|
||
// Select the minimal value among all rows
|
||
ret = min(ret, cms.matrix[idx])
|
||
}
|
||
return ret
|
||
}
|
||
|
||
// multiplyHigh64 implements (x * y) >> 64 "the long way" without access to a
|
||
// 128-bit type. This function is adapted from something similar in Tensorflow:
|
||
//
|
||
// https://github.com/tensorflow/tensorflow/commit/a47a300185026fe7829990def9113bf3a5109fed
|
||
//
|
||
// TODO(andrew-d): this could be replaced with a single "MULX" instruction on
|
||
// x86_64 platforms, which we can do if this ever turns out to be a performance
|
||
// bottleneck.
|
||
func multiplyHigh64(x, y uint64) uint64 {
|
||
x_lo := x & 0xffffffff
|
||
x_hi := x >> 32
|
||
buckets_lo := y & 0xffffffff
|
||
buckets_hi := y >> 32
|
||
prod_hi := x_hi * buckets_hi
|
||
prod_lo := x_lo * buckets_lo
|
||
prod_mid1 := x_hi * buckets_lo
|
||
prod_mid2 := x_lo * buckets_hi
|
||
carry := ((prod_mid1 & 0xffffffff) + (prod_mid2 & 0xffffffff) + (prod_lo >> 32)) >> 32
|
||
return prod_hi + (prod_mid1 >> 32) + (prod_mid2 >> 32) + carry
|
||
}
|
||
|
||
type mhValue[T any] struct {
|
||
count uint64
|
||
val T
|
||
}
|
||
|
||
// An minHeap is a min-heap of ints and associated values.
|
||
type minHeap[T any] []mhValue[T]
|
||
|
||
func (h minHeap[T]) Len() int { return len(h) }
|
||
func (h minHeap[T]) Less(i, j int) bool { return h[i].count < h[j].count }
|
||
func (h minHeap[T]) Swap(i, j int) { h[i], h[j] = h[j], h[i] }
|
||
|
||
func (h *minHeap[T]) Push(x any) {
|
||
// Push and Pop use pointer receivers because they modify the slice's length,
|
||
// not just its contents.
|
||
*h = append(*h, x.(mhValue[T]))
|
||
}
|
||
|
||
func (h *minHeap[T]) Pop() any {
|
||
old := *h
|
||
n := len(old)
|
||
x := old[n-1]
|
||
*h = old[0 : n-1]
|
||
return x
|
||
}
|